迈高陶瓷-山河智能股票行情
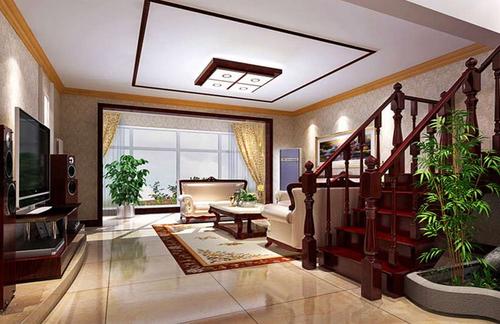
2023年9月14日发(作者:丰稷)
数据分析项⽬之:北京地区短租数据集分析及价格建模预测(天
池⼤数据竞赛)
项⽬名称:
北京地区短租数据集分析及价格建模预测
项⽬概述:
本项⽬主要是对短租数据集进⾏数据探索性分析,通过特征⼯程提取相关特征,在11个回归模型中对数据集进⾏建模训练,实现房价预测。
最后经过对⽐分析,选取其中表现较好的模型进⼀步调参优化,得到最优模型。
项⽬背景:
共享,通过让渡闲置资源的使⽤权,在有限增加边际成本的前提下,提⾼了资源利⽤效率。随着信息的透明化,越来越多的共享
发⽣在陌⽣⼈之间。短租,共享空间的⼀种模式,不论是否体验过⼊住陌⽣⼈的家中,你都可以从短租的数据⾥挖掘有趣的信息。
活动采⽤了短租房源相关的公开数据,包括了结构化的表格数据、⾮结构化的⽂本和地图数据。
项⽬流程:
1. 数据采集
2. 数据查看及预处理
3. EDA
4. 特征⼯程
5. 建模预测
6. 模型评估与优化
导包
1 import numpy as np
2 import pandas as pd
3 import seaborn as sns
4 import as plt
5 %matplotlib inline
6 import as ss
7 import missingno as miss
8 import jieba
9 from cessing import Normalizer
10 from cessing import StandardScaler,LabelEncoder
11 from cessing import OneHotEncoder,MinMaxScaler
12 from ors import KNeighborsRegressor
13 from _model import LinearRegression,Lasso,Ridge,LogisticRegression
14 from import DecisionTreeRegressor,ExtraTreeRegressor
15 from le import RandomForestRegressor,ExtraTreesRegressor
16 from le import AdaBoostRegressor,GradientBoostingRegressor
17 from s import mean_squared_error
18 from import SVR
19 from xgboost import XGBRegressor
20 from osition import PCA
21
22 from _selection import train_test_split
23 from _selection import KFold,StratifiedKFold
24 from _selection import GridSearchCV
2.查看数据概况
主要包括:查看数据类型、缺失值、异常值、数据分布情况等基本信息
1 display((),s)
数据情况如上图所⽰,主要是有关可租房源的信息,字段含义与英⽂含义基本⼀致。
字段含义:
id:样本ID
name:房屋名称
host_id:房东ID
host_name:房东姓名
neighbourhood_group:所属⾏政区
neighbourhood:所属⾏政区
latitude:纬度
longitude:经度
room_type:房间类型
price:价格
minimum_nights:最⼩可租天数
number_of_reviews:评论数量
last_review:最后⼀次评论时间
reviews_per_month:每⽉评论占⽐
calculated_host_listings_count:可出租房屋
availability_365:每年可出租时长
1 (data1) # 查看数据缺失情况
通过上图可以清楚的看到数据缺失情况。
1 () # 通过info()⽅法可以快速获取数据集的简单描述,特别是总⾏ 数、每个属性的类型和⾮空值的数量
1 be()
总结如下:
1. neighbourhood_group特征全部为空值;
2. name、last_review、reviews_per_month这⼏列存在数据缺失,稍后需要进⾏处理;
3. name、host_name、neighbourhood、room_type、last_review⼏个特征数据类型为String类型,后续可考虑量化处理;
4. room_type特征值存在重复现象,应该是⼀个分类特征。
5. 数据集包含28452条样本,共15个特征(price作为⽬标值)
6. price值:
最⼩值为0:说明可能存在异常值
25%分位值:235
中位数: 389
75%分位值:577
最⼤值: 68983,可能存在极值⼲扰
绘制各特征的直⽅图(可以看到数据的分布情况)
1 (bins=50,figsize=(20,15))
从上图可以看出很多特征分布呈长尾形,说明存在极值⼲扰,需要进⼀步处理
3. 特征⼯程(含EDA)
3.1 数据预处理:空值处理
1 # 创建数据表,分析数据缺失
2 def draw_missing_data_table(data):
3 total = ().sum().sort_values(ascending=False)
4 percent = (().sum()/().count()).sort_values(ascending=False)
5 missing_data = ([total,percent],axis=1,keys=['Total','Percent'])
6
7 return missing_data
1 null_ret = draw_missing_data_table(data1)
2 null_ret
由以上信息总结如下:
1. neighbourhood_group列全部为空 --- (删除此列)
2. last_review、reviews_per_month两列存在⽐较⼤的数据缺失 --- (填值)
3. name列只有⼀个空值,含义是房屋名称,⽆法进⾏填充 --- (删除)
1 # 删除neighbourhood_group列
2 (labels='neighbourhood_group',axis=1,inplace=True)
1 # name列
2 cond = data1['name'].isnull()
3 data1[cond] # 查看到name列只有⼀个空值,含义是房屋名称,⽆法进⾏填充,因此选择删除此条样本
1 # 删除name列空数据
2 cond = data1['name'].notnull()
3 data1 = data1[cond]
1 # last_review列空值
2 cond = data1['last_review'].isnull()
3 data1[cond] # 共有11157个空值
1 # 插值 0
2 data1['last_review'].fillna(0,inplace=True)
1 # reviews_per_month列空值
2 cond = data1['reviews_per_month'].isnull()
3 data1[cond] # 共11157个空值
1 mean = data1['reviews_per_month'].mean()
2 mean = round(mean,2) # 保留两位⼩数
3 mean
4
5 # 插值 mean
6 data1['reviews_per_month'].fillna(value=mean,inplace=True)
1 () # 查看已经不存在空值
⾃定义函数,规范neighbourhood列,使neighbourhood特征列的数据只包含中⽂名
1 def neighbourhood_str(data):
2 neighbourhoods = []
3 list = data['neighbourhood'].l('w+').tolist()
4 for i in list:
5 (i[0])
6 return neighbourhoods
7
8
9 data1['neighbourhood'] = neighbourhood_str(data1)
10 ()
3.2 探索性数据分析(EDA)
1 # 取出其中要分析的特征,单独观察各特征的数据分布情况
2 subsets = ['price','minimum_nights','number_of_reviews','reviews_per_month',
3 'calculated_host_listings_count','availability_365']
4
5
6
7 # 画出箱型图,观察数据集中的异常值
8
9 fig,ax = ts(len(subsets),1,figsize=(20,10))
10
11 ts_adjust(hspace=1) # 设置⼦图间的⾼度距离为100%
12 for i,subset in enumerate(subsets):
13 t(data1[subset],ax=ax[i],whis=2,orient='h')
14
1 # ⾃定义函数,展⽰观察数据集的各统计计量指标
2
3 '''
4 变异系数:标准差与平均数的⽐值,记为C·V。
5
6 1. 变异系数是衡量资料中各观测值变异程度的另⼀个统计计量。它可以消除单位和平均数不同对两个或对多个资料变异程度⽐较的影响。
7
8 2. 当进⾏两个或多个资料变异程度的⽐较时,如果度量单位与平均数相同,可以直接利⽤标准差来⽐较。如果度量单位和平均数不同时,
9 ⽐较其变异程度就不能采⽤标准差,⽽需采⽤标准差与平均数的⽐值来⽐较。
10
11 3. 计算公式:C·V =( 标准偏差 SD / 平均值Mean )× 100%
12
13 4. 在进⾏数据统计分析时,如果变异系数⼤于15%,则要考虑该数据可能不正常,应该删除。
14 '''
15
16 def EDA(df):
17 data = {}
18 for i in subsets:
19 ault(i,[]) # setdefault(key,default=None)如果键不存在于字典中,将会添加键并将值设为默认值
20 data[i].append(df[i].skew()) # 数据偏度
21 data[i].append(df[i].kurt()) # 数据峰度
22 data[i].append(df[i].mean()) # 数据均值
23 data[i].append(df[i].std()) # 数据⽅差
24 data[i].append(df[i].std()/df[i].mean()) # 变异系数
25 data[i].append(df[i].max()-df[i].min()) # 极值
26 data[i].append(df[i].quantile(0.25)) # 第⼀分位数 Q1
27 data[i].append(df[i].quantile(0.75)) # 第四分位数 Q3
28 data[i].append(df[i].quantile(0.75)-df[i].quantile(0.25)) # 四分位数间距IQR=Q3-Q1
29 data[i].append(df[i].median()) # 中位数
30
31 data_df = ame(data,index=['偏度','峰度','均值','标准差','变异系数','极差','第⼀分位数','第四分位数',
32 '四分位距','中位数'],columns=subsets)
33 return data_df.T
34
1 EDA(data1)
通过箱型图我们可以清晰的看到数据的异常值:
1. 因为是短租场景,价格price中存在上万的租⾦可能就是异常值;
2. 最少租住天数也存在很多异常值。
异常值处理
1 # 删除price特征中的异常值(删除价格为0的数据)
2 cond = data1['price'] > 0
3 data1 = data1[cond]
4
特征选择1
1 # 剔除与分析⽬标不相关的属性 latitude,longitude
2 # 对于短租来说,在⼀个地区⾥,价格差距不会太⼤,⼀般会和房⼦类型等其他因素有关
3
4 (labels=['latitude','longitude'],axis=1,inplace=True)
1 # 使⽤ sns的 violinplot()函数绘制⼩提琴图,查看价格集中在哪个阶段
2
3 (figsize=(15,6))
4 t(1,2,1)
5 plot(data1['price'])
6
7 t(1,2,2)
8 plot((data1['price'])) # 使⽤log函数对特征进⾏平滑处理
观察⼩提琴图可以发现:
1. 数据⽐较集中,只存在极少部分⾼价格数据;
2. 使⽤log函数对特征进⾏平滑处理后,将价格之间的差距缩⼩,得到了⼀个更合理的分布图。
特征选择2
1 # 对价格进⾏单独分析,观察价格的具体分布
2
3 ot(data1[data1['price'] < 3000]['price'])
观察上图可以发现:
[0,1000]区间内的数据分布接近正态分布,在预测价格时取此区间数据进⾏建模即可。
1 # 取出价格在[0,1000]区间的数据
2
3 data2 = data1[data1['price'] <= 1000]
4 # (25977, 13)
查看房源总体分布情况
1 # 查看房源总体分布情况
2
3 # data2['neighbourhood'].value_countsounts() # AttributeError: 'Series' object has no attribute 'value_countsounts'
4 listing = _counts() # 这种写法就不会报错
5 listing = ame(listing)
6 listing['percent'] = (listing['neighbourhood'].values/()).round(3)
7 listing
1 # 绘制饼图,查看总体分布情况
2
7 (figsize=(15,15))
8 ('各区listing分布占⽐',fontdict={'fontsize':18})
9
10 # 朝阳区、海淀区、东城区3个占⽐较⾼的区使⽤ explode突出显⽰
11 (listing,
12 labels=labels,
13 autopct='%0.2f%%',
14 explode=[0.07 if i in ['东城区','朝阳区','海淀区'] else 0 for i in labels],
15 startangle=90,
16 counterclock=False,
17 textprops={'fontsize':10,'color':'black'},
18 colors=_palette('summer_r',n_colors=18))
19
20 (loc='best',shadow=True,fontsize=11)
通过上图可以知道,房源整体分布中,朝阳、海淀、东城三区占⽐较⼤,其他各区占⽐较⼩
1 # 对各区房源价格均值进⾏对⽐(透视表)
2
3 price_pair = _table(data2,index='neighbourhood',columns='room_type',
4 values='price',aggfunc=)
5
6 price_pair
1 # 将最终结果进⾏可视化展⽰(热图)
2
3 (figsize=(12,12))
4 p(price_pair,cmap=_palette('PiYG',n_colors=32),annot=True,fmt='.0f')
通过观察发现:
1. Entire home/apt类型:东城、密云、平⾕、延庆、怀柔区价格较⾼;
2. Private room类型:延庆、怀柔、门头沟区价格较⾼;
3. Shared room类型:延庆、怀柔区价格较⾼。
总体延庆县和怀柔区价格较⾼。
绘制词云图
1 # 分析受关注程度⾼的房源与受关注程度低的房源之间关于名字属性有什么区别
2
3 '''获取各区评论数量top或者bottom的数量,根据 num 参数获取排名靠前的数据以及排名靠后的数据'''
4 def get_review_tb(df,num):
5 result = []
6 groups = y('neighbourhood')
7 for x,group in groups:
8 if num>0:
9 (_values(by='number_of_reviews',ascending=False)[:num])
10 if num<0:
11 (_values(by='number_of_reviews',ascending=False)[num:])
12 result = (result)
13
14 return result
15
1 reviews_top10 = get_review_tb(data2,10)
2 reviews_bottom10 = get_review_tb(data2,-10)
3
4 display(reviews_(),reviews_())
1 # 打印停⽤词词汇表
2
3 with open('./','rt',encoding='utf8') as f:
4 result = ().split()
5
6 print(result)
1 # 导⼊相关分析包
2
3 import jieba
4 from wordcloud import WordCloud
5 from imageio import imread
1 '''获取房源名字中的关键字'''
2
3 def get_words(df):
4 s = []
5 words_dic = {}
6 with open('./','rt',encoding='utf8') as f: # 根据停⽤词过滤词汇
7 result = ().split()
8 for i in df:
9 words = (i) # 利⽤jieba对房屋名称进⾏拆词分析,获取⾼频词汇
10 word = [x for x in words if x not in result]
11 (word)
12 for word in s:
13 if word != ' ': # 去掉字符串为空格的数据
14 words_ault(word,0) # 为该字典设置默认值,如果不存在则添加,如果该键存在,则跳过并忽略
15 words_dic[word] += 1
16
17 return words_dic,s
18
19
20
21 '''删除⽆关字符'''
22
23 def select_word(dic):
24 new_dic = {}
25 for key,val in ():
26 if key not in ['。','、',',','⼁']:
27 if val > 6:
28 new_dic[key] = val
29
30 return new_dic
top_words,s1 = get_words(reviews_('str')) # 强制转化为字符串格式
bottom_words,s2 = get_words(reviews_('str')) # 强制转化为字符串格式
# 转换成Series,⽅便绘图可视化
top_words_s = (select_word(top_words)).sort_values(ascending=False)
bottom_words_s = (select_word(bottom_words)).sort_values(ascending=False)
print(top_words_s)
print('*'*100)
print(bottom_words_s)
1 # 绘图进⾏⽐较,受关注度较⼩和受关注度较⼤的房源名称中词的分布情况
2
3 fig,ax = ts(2,1,figsize=(10,5))
4 ax[0].set_title('受关注较⼤房源信息⾥name中词的出现次数分布图')
5 ax[1].set_title('受关注较⼩房源信息⾥name中词的出现次数分布图')
6
7 # (top_words_s)
8 top_words_(kind='bar',ylim=[0,40],color='r')
9 bottom_words_(kind='bar',ylim=[0,40],color='r')
观察上图发现:
1. ⽆论是受关注⼩还是受关注⼤的房源,⼤部分词汇都集中在地铁、公寓、北京、温馨等词汇中;
2. 总体来看,受关注程度与房屋描述相关性不⼤。
1 '''绘制词云图'''
2
3 def cloud_s(datas):
4 new_data = []
5 for data in datas:
6 if data != ' ':
7 if data not in ['。','、',',','⼁']:
8 new_(data)
9
10 return new_data
1 s1 = cloud_s(s1)
2 s1 = ' '.join(s1)
3 s1
1 s2 = cloud_s(s2)
2 s2 = ' '.join(s2)
3 s2
1 mask= imread('./') # 此处为使⽤遮罩的情况,即⽣成的词云形状
2
3 def draw_cloude(mask, s, num):
4 wordcloud = WordCloud(background_color = '#FFFFFF', # 词云图⽚的背景颜⾊
5 width = 800, # 词云图⽚的宽度,默认400像素
6 height = 800, # 词云图⽚的⾼度,默认200像素
7 font_path = '/opt/anaconda3/lib/python3.8/site-packages/wordcloud/', # 词云指定字体⽂件的完整路径
8 # max_words = 200, #词云图中最⼤词数,默认200
9 # max_font_size = 80, # 词云图中最⼤的字体字号,默认None,根据⾼度⾃动调节
10 # min_font_size = 20, # 词云图中最⼩的字体字号,默认4号
11 font_step = 1, # 词云图中字号步进间隔,默认1
12 mask = mask, # 词云形状,默认None,即⽅形图
13 ).generate(s) # 由txt⽂本⽣成词云
14 #返回对象
15 image_produce = _image()
16 #显⽰图像
17 # image_()
18 # 将词云图保存为名为sample的⽂件
19 _file("sample%" % num)
20
21
22 draw_cloude(mask, s1, 1)
23 draw_cloude(mask, s2, 2)
1 sample1 = ('./')
2 (sample1)
3
4
5 sample2 = ('./')
6 (sample2)
效果如图:
3.2 数据转换
name、host_name、neighbourhood、room_type、last_review⼏个特征数据类型为String类型。其中:
1. neighbourhood、room_type是对price(价格)有较⼤影响的特征,选择量化处理;
2. last_review是时间数据,选择转换为时间类型或删除
3.2.1 neighbourhood、room_type量化处理
1 # LabelEncoder
2 le = LabelEncoder()
3
4 labels = ['neighbourhood','room_type']
5 for col in labels:
6 data2[col] = _transform(data2[col])
7
8 ()
3.2.2 剔除 last_review、reviews_per_month 两个特征
# 剔除 last_review、reviews_per_month 两个特征
drop_labels = ['last_review','reviews_per_month']
(labels=drop_labels,axis=1,inplace=True)
特征选择3
1 # 查看各特征之间相关性系数
2 # 相关性系数越靠近 1 或 -1 ,则代表特征之间越有相关性
3 pair_cols = ['price', 'neighbourhood', 'room_type', 'minimum_nights', 'number_of_reviews',
4 'calculated_host_listings_count', 'availability_365']
5
6 correlation = data2[pair_cols].corr()
7
8 correlation
1 # 可视化
2
3 (figsize=(12,12))
4 ot(data2[pair_cols])
1 other_feature = data2[['id','name','host_id','host_name']] # ID等属性先取出来,⽅便后⾯合并表格使⽤
2
3 # 删除['id','name','host_id','host_name']特征
4 data3 = (['id','name','host_id','host_name'],axis=1)
1 ()
观察上图可以看到,房屋类型和价格相关性最⾼(-0.495083),其他特征或多或少有⼀定相关性
4. 建模分析---多种回归模型下简单预测的⽐较分析
⼀:不做处理
x_train, x_test, y_train, y_test = train_test_split(x_dum, y, test_size = 0.25, random_state = 1)
⼆:尝试平滑处理预测值y,即平滑处理y值,x不处理。(x代表特征,y代表预测值)
y_log = (y)
x_train, x_test, y_train_log, y_test_log = train_test_split(x_dum,y_log,test_size = 0.25,random_state = 1)
三:再整理出⼀组标准化的数据,通过对⽐可以看出模型的效果有没有提⾼
1 # 数据
2 X = [:,[0,1,3,4,5,6]]
3
4 # ⽬标值
5 y = [:,2]
1 # 哑编码
2 one = OneHotEncoder()
3
4 X = _transform(X)
⼀、不做处理
1 # 数据分割
2 X_train,X_test,y_train,y_test = train_test_split(X,y,test_size=0.2)
1 # 创建模型对象
2 models=[LinearRegression(),KNeighborsRegressor(),SVR(),Ridge(),Lasso(),DecisionTreeRegressor(),
3 ExtraTreeRegressor(),XGBRegressor(),RandomForestRegressor(),AdaBoostRegressor(),GradientBoostingRegressor()]
4
5 models_str=['LinearRegression','KNNRegressor','SVR','Ridge','Lasso','DecisionTree',
6 'ExtraTree','XGBoost','RandomForest','AdaBoost','GradientBoost']
1 # 循环出每⼀个模型,进⾏模型的构建
2
3 big_score = []
4 big_mean_score = []
5
6 for name,model in zip(models_str,models):
7 score_ = []
8 mean_score = []
9 print('开始训练模型:' + name)
10 model = model #建⽴模型
11 (X_train, y_train)
12 y_pred = t(X_test)
13 score = (X_test, y_test)
14 score_.append(str(score)[:5])
15 mean_(mean_squared_error(y_test, y_pred))
16
17 big_(score_)
18 big_mean_(mean_score)
19 print(name +' 得分:'+str(score))
20 print('均⽅误差:',mean_squared_error(y_test, y_pred))
1 df = ame((big_score).reshape(-1,1),index=models_str, columns=['score'])
2 df['mean_score'] = (big_mean_score).reshape(-1,1)
3 df
通过观察结果:
1. DecisionTree、ExtraTree、AdaBoost得分最低,SVR得分也不⾼,原因是SVR训练数据需要标准化处理;
2. XGBoost、RandomForest、GradientBoost得分较⾼,这⼏个都是集成算法,后续对其进⾏参数调优,效果应该更好。
⼆:尝试平滑处理预测值y,即平滑处理y值,x不处理。
1 # 平滑处理
2 y_log = (y)
3
4 # 数据分割
5 X_train,X_test,y_train_log,y_test_log = train_test_split(X,y_log,test_size=0.2)
1 # 循环出每⼀个模型,进⾏模型的构建
2
3 big_score = []
4 big_mean_score = []
5
6 for name,model in zip(models_str,models):
7 score_ = []
8 mean_score = []
9 print('开始训练模型:' + name)
10 model = model #建⽴模型
11 (X_train, y_train_log)
12 y_pred = t(X_test)
13 score = (X_test, y_test_log)
14 score_.append(str(score)[:5])
15 mean_(mean_squared_error(y_test_log, y_pred))
16
17 big_(score_)
18 big_mean_(mean_score)
19 print(name +' 得分:'+str(score))
20 print('均⽅误差:',mean_squared_error(y_test_log, y_pred))
1 df_log = ame((big_score).reshape(-1,1),index=models_str, columns=['score_log'])
2 df_log['mean_score_log'] = (big_mean_score).reshape(-1,1)
3 df_log
通过观察结果:
1. 部分模型预测效果得到了很好的提升;
2. XGBoost有很明显的提升。
三:整理出⼀组标准化的数据,通过对⽐可以看出模型的效果有没有提⾼
1 # 对数据进⾏标准化处理后,再进⾏训练
2
3 scale_X = StandardScaler(with_mean=False)
4 X1 = scale__transform(X)
5 scale_y = StandardScaler()
6 y = (y).reshape(-1,1)
7 y1 = scale__transform(y)
8 y1 = () # 扁平化数据
9 X_train1, X_test1, y_train1, y_test1 = train_test_split(X1, y1, test_size =0.2)
1 # 循环出每⼀个模型,进⾏模型的构建
2
3 big_score_reg = []
4 big_mean_score_reg = []
5
6 for name,model in zip(models_str,models):
7 score_ = []
8 mean_score = []
9 print('开始训练模型:' + name)
10 model = model #建⽴模型
11 (X_train1, y_train1)
12 y_pred = t(X_test1)
13 score = (X_test1, y_test1)
14 score_.append(str(score)[:5])
15 mean_(mean_squared_error(y_test1, y_pred))
16
17 big_score_(score_)
18 big_mean_score_(mean_score)
19 print(name +' 得分:'+str(score))
20 print('均⽅误差:',mean_squared_error(y_test1, y_pred))
1 df_reg = ame((big_score_reg).reshape(-1,1),index=models_str, columns=['score_reg'])
2 df_reg['mean_score_reg'] = (big_mean_score_reg).reshape(-1,1)
3 df_reg
通过观察结果:
1 # 先对n_estimators参数进⾏调试
2
3 cv_params = {'n_estimators':[i for i in range(250,300)]}
4 other_params = {'learning_rate': 0.1, 'n_estimators': 500, 'max_depth': 5, 'min_child_weight': 1, 'seed': 0,
5 'subsample': 0.8, 'colsample_bytree': 0.8, 'gamma': 0, 'reg_alpha': 0, 'reg_lambda': 1}
6
7 xgb = XGBRegressor(**other_params)
8 clf = GridSearchCV(estimator=xgb, param_grid=cv_params, scoring='r2', cv=5, verbose=1, n_jobs=4)
9 (X_train,y_train_log)
10 evalute_result = _results_
11
12 print('每轮迭代运⾏结果:{0}'.format(evalute_result))
13 print('参数的最佳取值:{0}'.format(_params_))
14 print('最佳模型得分:{0}'.format(_score_))
观察结果可知:
n_estimators 最好参数为 273,更新参数,继续调参
1 # 优化max_depth和min_child_weight参数
2
3 cv_params = {'max_depth': [3, 4, 5, 6, 7, 8, 9, 10], 'min_child_weight': [1, 2, 3, 4, 5, 6]}
4
5 other_params = {'learning_rate': 0.1, 'n_estimators': 273, 'max_depth': 5, 'min_child_weight': 1, 'seed': 0,
6 'subsample': 0.8, 'colsample_bytree': 0.8, 'gamma': 0, 'reg_alpha': 0, 'reg_lambda': 1}
7
8 xgb = XGBRegressor(**other_params)
9 clf = GridSearchCV(estimator=xgb, param_grid=cv_params, scoring='r2', cv=5, verbose=1, n_jobs=4)
10 (X_train,y_train_log)
11 evalute_result = _results_
12
13 print('每轮迭代运⾏结果:{0}'.format(evalute_result))
14 print('参数的最佳取值:{0}'.format(_params_))
15 print('最佳模型得分:{0}'.format(_score_))
观察结果可知:
max_depth 最好参数为 9,min_child_weight 最好参数为 2,更新参数,继续调参
1 # 进⼀步优化gamma
2
3 cv_params = {'gamma': [0.1, 0.2, 0.3, 0.4, 0.5, 0.6]}
4
5 other_params = {'learning_rate': 0.1, 'n_estimators': 273, 'max_depth': 9, 'min_child_weight': 2, 'seed': 0,
6 'subsample': 0.8, 'colsample_bytree': 0.8, 'gamma': 0, 'reg_alpha': 0, 'reg_lambda': 1}
7
8 xgb = XGBRegressor(**other_params)
9 clf = GridSearchCV(estimator=xgb, param_grid=cv_params, scoring='r2', cv=5, verbose=1, n_jobs=5)
10 (X_train, y_train_log)
11 evalute_result = _results_
12 print('每轮迭代运⾏结果:{0}'.format(evalute_result))
13 print('参数的最佳取值:{0}'.format(_params_))
14 print('最佳模型得分:{0}'.format(_score_))
观察结果可知:
gamma 最好参数为 0.1,更新参数,继续调参
1 # 优化subsample 和 colsample_bytree
2
3 cv_params = {'subsample': [0.6, 0.7, 0.8, 0.9], 'colsample_bytree': [0.6, 0.7, 0.8, 0.9]}
4
5 other_params = {'learning_rate': 0.1, 'n_estimators': 273, 'max_depth': 9, 'min_child_weight': 2, 'seed': 0,
6 'subsample': 0.8, 'colsample_bytree': 0.8, 'gamma': 0.1, 'reg_alpha': 0, 'reg_lambda': 1}
7
8 xgb = XGBRegressor(**other_params)
9 clf = GridSearchCV(estimator=xgb, param_grid=cv_params, scoring='r2', cv=5, verbose=1, n_jobs=5)
10 (X_train, y_train_log)
11 evalute_result = _results_
12 print('每轮迭代运⾏结果:{0}'.format(evalute_result))
13 print('参数的最佳取值:{0}'.format(_params_))
14 print('最佳模型得分:{0}'.format(_score_))
观察结果可知:
colsample_bytree 最好参数为 0.8,subsample 最好参数为 0.9,更新参数,继续调参
1 # 优化 reg_alpha 和 reg_lambda
2
3 cv_params = {'reg_alpha': [0.05, 0.1, 0.2, 0.5, 1], 'reg_lambda': [0.01, 0.05, 0.1, 1, 2, 3]}
4
5 other_params = {'learning_rate': 0.1, 'n_estimators': 273, 'max_depth': 9, 'min_child_weight': 2, 'seed': 0,
6 'subsample': 0.9, 'colsample_bytree': 0.8, 'gamma': 0.1, 'reg_alpha': 0, 'reg_lambda': 1}
7
8 xgb = XGBRegressor(**other_params)
9 clf = GridSearchCV(estimator=xgb, param_grid=cv_params, scoring='r2', cv=5, verbose=1, n_jobs=5)
10 (X_train, y_train_log)
11 evalute_result = _results_
12 print('每轮迭代运⾏结果:{0}'.format(evalute_result))
13 print('参数的最佳取值:{0}'.format(_params_))
14 print('最佳模型得分:{0}'.format(_score_))
观察结果可知:
reg_alpha 最好参数为 1,reg_lambda 最好参数为 0.05,更新参数,继续调参
1 # 优化 learning_rate
2
3 cv_params = {'learning_rate': [0.01, 0.05, 0.07, 0.1, 0.2]}
4
5 other_params = {'learning_rate': 0.1, 'n_estimators': 273, 'max_depth': 9, 'min_child_weight': 2, 'seed': 0,
6 'subsample': 0.9, 'colsample_bytree': 0.8, 'gamma': 0.1, 'reg_alpha': 1, 'reg_lambda': 0.05}
7
8 xgb = XGBRegressor(**other_params)
9 clf = GridSearchCV(estimator=xgb, param_grid=cv_params, scoring='r2', cv=5, verbose=1, n_jobs=5)
10 (X_train, y_train_log)
11 evalute_result = _results_
12 print('每轮迭代运⾏结果:{0}'.format(evalute_result))
13 print('参数的最佳取值:{0}'.format(_params_))
14 print('最佳模型得分:{0}'.format(_score_))
观察结果可知:
learning_rate 最好参数为 0.1
1 # 整合所有的参数,进⾏训练
2
3 other_params = {'learning_rate': 0.1, 'n_estimators': 273, 'max_depth': 9, 'min_child_weight': 2, 'seed': 0,
4 'subsample': 0.9, 'colsample_bytree': 0.8, 'gamma': 0.1, 'reg_alpha': 1, 'reg_lambda': 0.05}
5
6 print('开始训练模型')
7 # 建模
8 xgb = XGBRegressor(learning_rate=0.1, n_estimators=273, max_depth=9, min_child_weight=2, seed=0,
9 subsample=0.9, colsample_bytree=0.8, gamma=0.1, reg_alpha= 1, reg_lambda=0.05)
10 (X_train,y_train_log)
11 y_pre = t(X_test)
12 score = (X_test,y_test_log)
13
14 print('得分:' + str(score))
15 print('均⽅误差:',mean_squared_error(y_test_log,y_pre))
6. 总结
最终得到的结果虽然有提升,但得分并不算⾼,分析原因有:
1. 数据集特征太少;
2. 数据集特征与价格特征之间的相关性⽐较⼩。
北京万科朗润园-A股的分红就是一个骗局
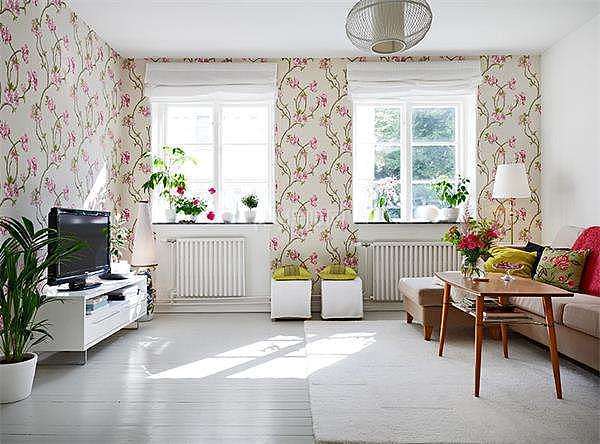
更多推荐
北京一个月短租
发布评论